During 2020-2022, OARC received funding from NSF and NIST to conduct a three-workshop symposium titled “Strategy for Resilient Manufacturing Ecosystems Through Artificial Intelligence (AI)” on the opportunities and challenges for artificial intelligence (AI) as applied to manufacturing. The symposium brought together industry, academia, and government experts to explore the potential roles of each sector in performing R&D as well as ideas for how to implement AI in manufacturing, which could serve to inform the development of the U.S. Advanced Manufacturing strategic plan.
Outcomes and Recommendations
In recognizing that AI value depends on company and intercompany strategies, the Symposium addressed factory and inter-company solutions in conjunction with industry strategies for scaling access to data and expertise that are largely available only through collaboration. R&D about industry scaling and deployment is required to push these capabilities to manufacturing with pre-production prototypes and initial production trials. However, technology R&D from concept through pre-production is not sufficient to initiate AI deployment at scale. Symposium recommendations address this issue with a roadmap organized to overcome the current lack of industry tools, trust, confidence, and experience with industry R&D focused on the barriers to AI scaling and deployment.
There are eight specific recommendations for collaborative actions by industry, government, and academia:
- Establish new and/or expanded Public Private Partnerships (PPPs) to facilitate a broad range of R&D.
- Research, develop, and demonstrate advanced software tools, models, and infrastructure for AI/ML implementation and scale-up in manufacturing.
- Establish programs that achieve industry collaboration on an integrated and trusted set of shared capabilities.
- Initiate R&D to enable industry-wide scaling and deployment of AI applications in manufacturing.
- Educate and train a digital-savvy manufacturing workforce with software and hardware tools needed to deploy and scale the use of data and AI with trust and confidence.
- Enable digital capabilities at small and medium-sized manufacturers (SMMs).
- Incentivize AI adoption throughout established supply chains.
- Promote new business models for AI adoption.
As this adoption cycle takes hold, the market-driven forces of entrepreneurship and investment capital will ultimately lead to industry-wide adoption of AI technology, and the U.S. manufacturing industry will be on its way to achieving global leadership and resilient supply chains.
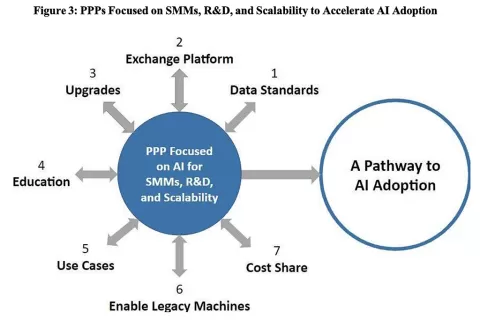
The workshop proposed seven PPPs for a pathway to AI adoption:
- Data Standards
- Exchange Platform
- Upgrades
- Education
- Use Cases
- Enable Legacy Machines
- Cost Share
Workshop Reports
The outcomes of each workshop are documented in three separate reports linked below:
The final report from the grant can be found on the NIST website.
Learn More
In 2020, the National Science and Technology Council (NSTC) Subcommittee on Advanced Manufacturing and Subcommittee on Machine Learning and Artificial Intelligence articulated cross-agency interest in the value and timeliness of organizing a symposium to outline benefits of AI adoption in manufacturing and identify issues that inhibit wide scale adoption. The National Science Foundation (NSF) and the National Institute of Standards and Technology (NIST) funded OARC to host the “Strategy for Resilient Manufacturing Ecosystems Through Artificial Intelligence (AI)” symposium, which took place in three workshops between 2020 and 2022.
AI in manufacturing operations includes software systems that can recognize, simulate, predict, and optimize manufacturing process conditions, and human and machine actions that impact production. AI in manufacturing also includes software systems that support supply chain logistics and the business and management processes needed to establish and sustain operational AI systems, including mechanisms for the secure and trustworthy exchange and sharing of the data that fundamentally powers them. Lastly, AI in manufacturing includes software systems that have the capability to search, discover, clean, augment, access, and categorize data obtained from diverse and widely distributed networked resources for use in training AI solutions that target specific classes of manufacturing challenges faced by industry. AI in manufacturing depends in large part on research to move beyond current machine learning architectures that are optimized for image recognition to architectures specifically suited to categorizing the data generated by manufacturing machines, equipment, processes, and operations.
The high potential for pervasive application of AI in manufacturing to provide a world-leading advantage to the U.S. manufacturing industry justifies a much faster pace of development of the skills and tools needed to accelerate industry adoption. The full potential of AI accrues from industry-wide actions that are orchestrated to encourage adoption. Realizing the full potential of AI requires the adoption of new business practices that enable the industry to become networked and interconnected (a.k.a. Smart Manufacturing). Innovative technologies, services, infrastructure, and software tools are needed to provide manufacturers assurance that their valued data will be protected when selected data is aggregated industry-wide to provide the enhanced benefits that AI/ML methods can provide at scale. Those benefits include new network-based business models that provide faster process development and increased productivity, quality, and environmental sustainability. It is also critical to ensure that strong collaborations between the AI and manufacturing communities are fostered (including overcoming cultural and language barriers) to achieve the goals identified by the Symposium.