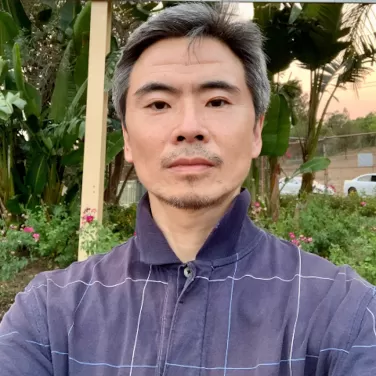
Qiyang Hu
Qiyang currently works as a computational and data scientist in the Computational Science Group in the Office of Advanced Research Computing at UCLA.
As a mechanical engineering Ph.D. (UCLA, 2006), Qiyang’s educational background is to numerically solve physics problems dominated by partial differential equations using high-performance computational techniques. His research experience includes mathematical modeling/simulation of different physics systems, such as stress/structure analysis and chemical reaction kinetics.
With the active and rapid developments occurring in machine learning (ML) and deep learning (DL) technologies, in recent years Qiyang focuses his main interests on exploiting the possibilities of leveraging his high-performance computing and large-scale simulation expertise in data science and data analytics. Specifically, he is actively involved in the following activities:
- Offering a year-long series of classes in the form of quarterly OARC/IDRE workshops. The class topics cover high-performance python, the machine learning toolbox Scikit-Learn, introductory deep learning, and practical uses of the machine learning framework PyTorch.
- Close-tracking the deep-learning technology developments, including graph neural networks, GAN-based generative techniques, transformer-based deep learning models, self-supervised learning, and the integration of deep learning and causal inference.
- Exploring deep-learning applications in scientific modeling and computation: e.g. data-driven modeling, deep-learning-based surrogate modeling, differentiable physics, deep Galerkin methods, etc.
- Experimenting with large-scale deep-learning models on Hoffman2 Cluster and cloud-based HPC clusters to build practice prototypes.
Qiyang's goal is to build his knowledge base through literature reviews and research in order to keep up with the latest trends and developments in ML/DL related fields and to deepen our understanding of the capabilities and strengths, as well as limitations and challenges, of deep learning technologies so that he can provide sustainable support to UCLA faculty and staff to employ statistical-method-based data analytics and modeling to perform fundamental research as well as to improve the efficacy and efficiency of their research activities.